
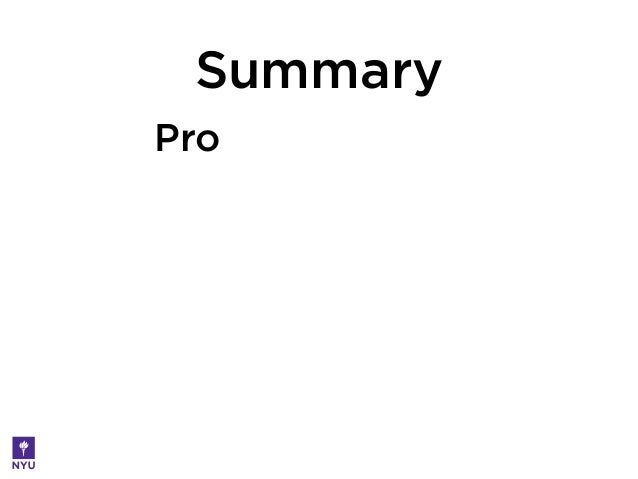
Recent advances in automated scoring often leverage textual representations based on pre-trained language models such as BERT and GPT as input to scoring models. We evaluate our framework on a real-world dataset of student responses to open-ended math questions and show that our framework (often significantly) outperforms existing approaches, especially for new questions that are not seen during training.Īutomated scoring of open-ended student responses has the potential to significantly reduce human grader effort. Second, we use an in-context learning approach that provides scoring examples as input to the language model to provide additional context information and promote generalization to previously unseen questions. First, we use MathBERT, a variant of the popular language model BERT adapted to mathematical content, as our base model and fine-tune it for the downstream task of student response grading. In this paper, we study the problem of automatic short answer grading for students' responses to math questions and propose a novel framework for this task. However, these approaches have several key limitations, including i) they use pre-trained language models that are not well-adapted to educational subject domains and/or student-generated text and ii) they almost always train one model per question, ignoring the linkage across a question and result in a significant model storage problem due to the size of advanced language models. Current state-of-the-art approaches use neural language models to create vectorized representations of students responses, followed by classifiers to predict the score.
ELECTRONIC ESSAY GRADER HOW TO
Parallel to these advances, the focus of technology-based assessment has shifted from an individual and summative approach to one which is cooperative, diagnostic and more learning-centred to implement efficient testing for personalised learning.Īutomatic short answer grading is an important research direction in the exploration of how to use artificial intelligence (AI)-based tools to improve education. Developments in IT have made it possible to design different assessments, thus boosting the number of ways students can demonstrate their skills and abilities. Recent work has focused on logfile analysis, educational data mining and learning analytics. A systematic review of media studies was conducted to detect these effects the results were varied.
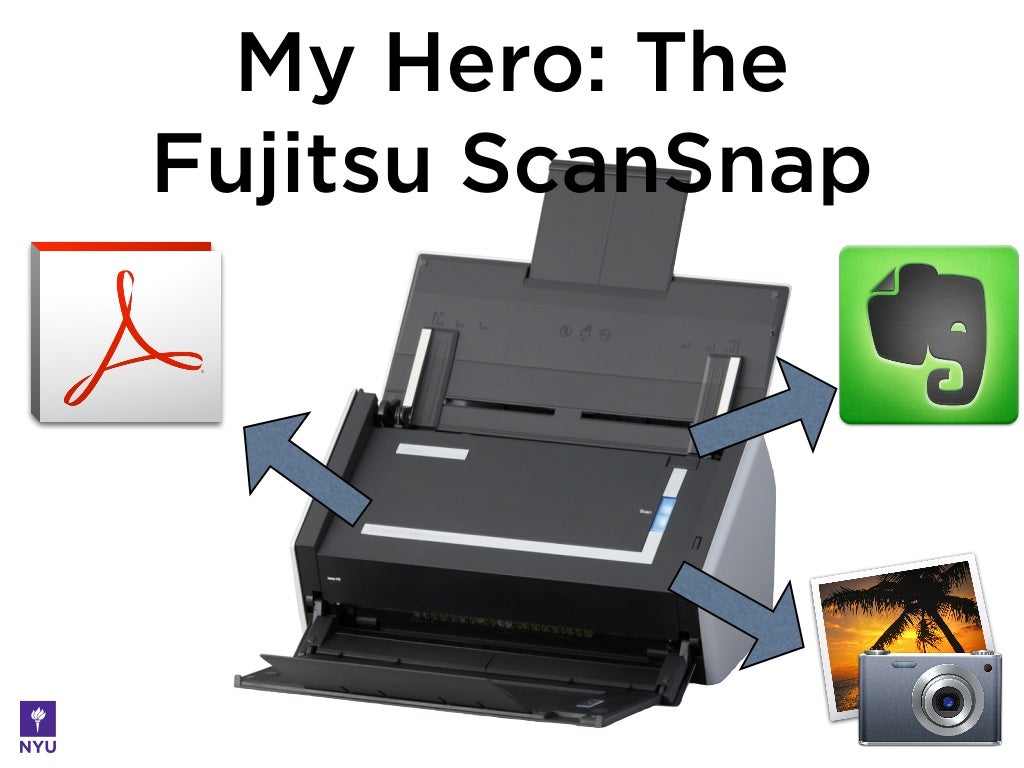
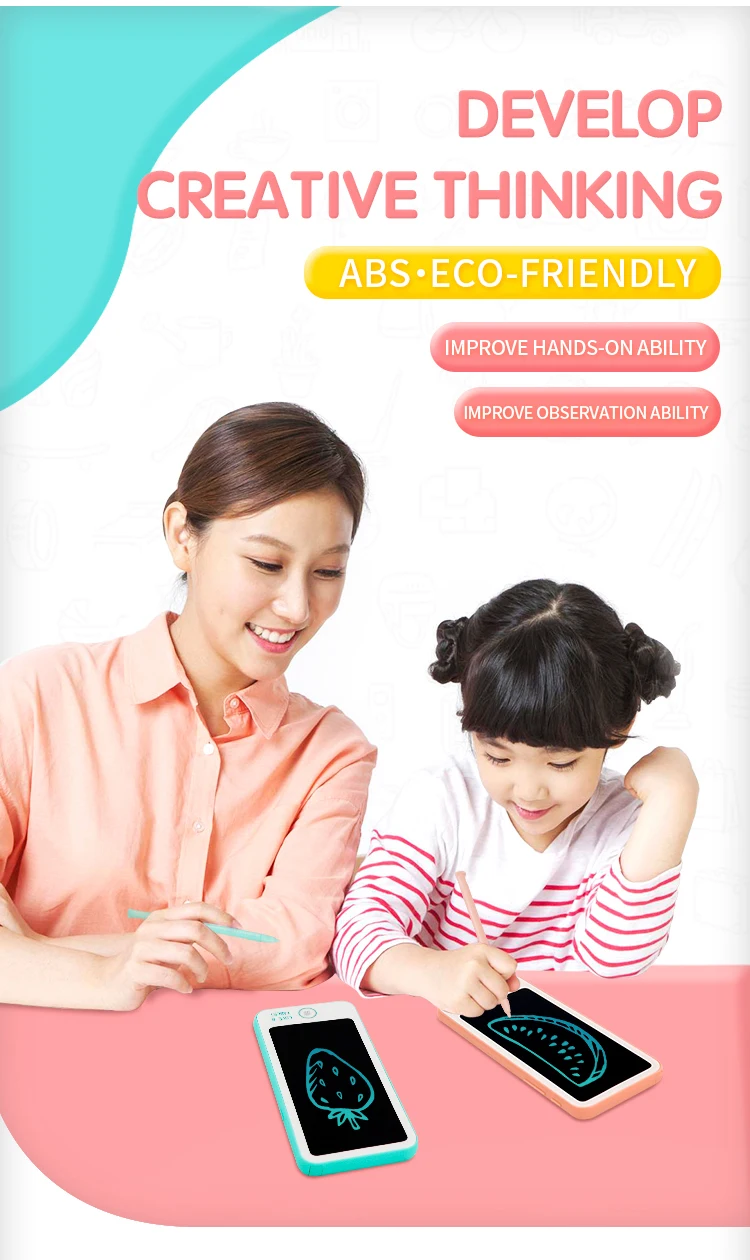
Around the turn of the millennium, studies centred on computer-based and paper-and-pencil test comparability to ascertain the effect of delivery medium on students' test achievement. Developments in technology-based assessment stretch back three decades. This paper presents developmental trends in technology-based assessment in an educational context and highlights how technology-based assessment has reshaped the purpose of educational assessment and the way we think about it. The possibilities, advantages and challenges of TBA are growing in accordance with the level of application (e.g., item development, delivery, scoring and feedback), type of technology (e.g., desktop computer, touchscreen tablets and eye-tracking technologies), methodology used (e.g., fixed testing or adaptive testing), delivery (e.g., internet-based, local server delivery and delivery on removable media), scoring (e.g., automatic, computer-based (CB), but not automatic, human scoring item-level scoring based on the actual answer of the students or logfile and process data analyses based on the actions of the students), item types (e.g., traditional multiple-choice or state-of-the-art third-generation innovative item types, including interactivity), domains (e.g., domains can be assessed using traditional methods, such as reading fixed texts, or domains requiring TBA, such as reading digital and printed texts) and the technological conditions of the assessment.
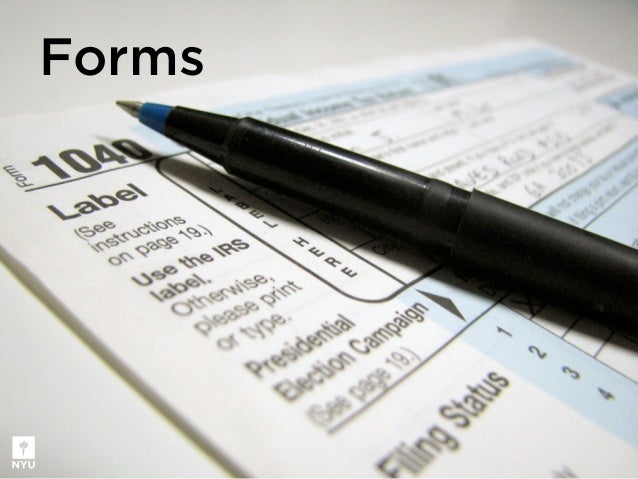
The use of technology in assessment may lead to improved assessment, thus offering numerous advantages (e.g., automatic item generation, presenting dynamic stimuli and automatic scoring Becker, 2004 Csapó et al., 2014 Dikli, 2006 Mitchell et al., 2002 Valenti et al., 2003), cutting costs (e.g., delivery, distributing results and evaluating answers Bennett, 2003 Christakoudiset al., 2011 Wise and Plake, 1990) and laying the groundwork for new innovations (e.g., measuring new constructs and using new item types Dörner and Funke, 2017 Pachler et al., 2010) in educational assessment.
